Machine Learning & Data Science
Machine learning (ML), the development and study of computer algorithms that learn from data, is increasingly important across a wide array of applications, from virtual personal assistants to social media and product recommendation systems. ML methods have also driven key developments in the natural sciences: virtual screening of drug-like molecules for medical applications, rapid prediction of physical data, and computer-aided synthesis planning have all been facilitated by ML. The development of ML tools for synthetic methodology development and catalysis could enable chemists to make data-efficient choices and learn from that data in the course of reaction prediction, reaction condition optimization, and mechanistic interrogation.
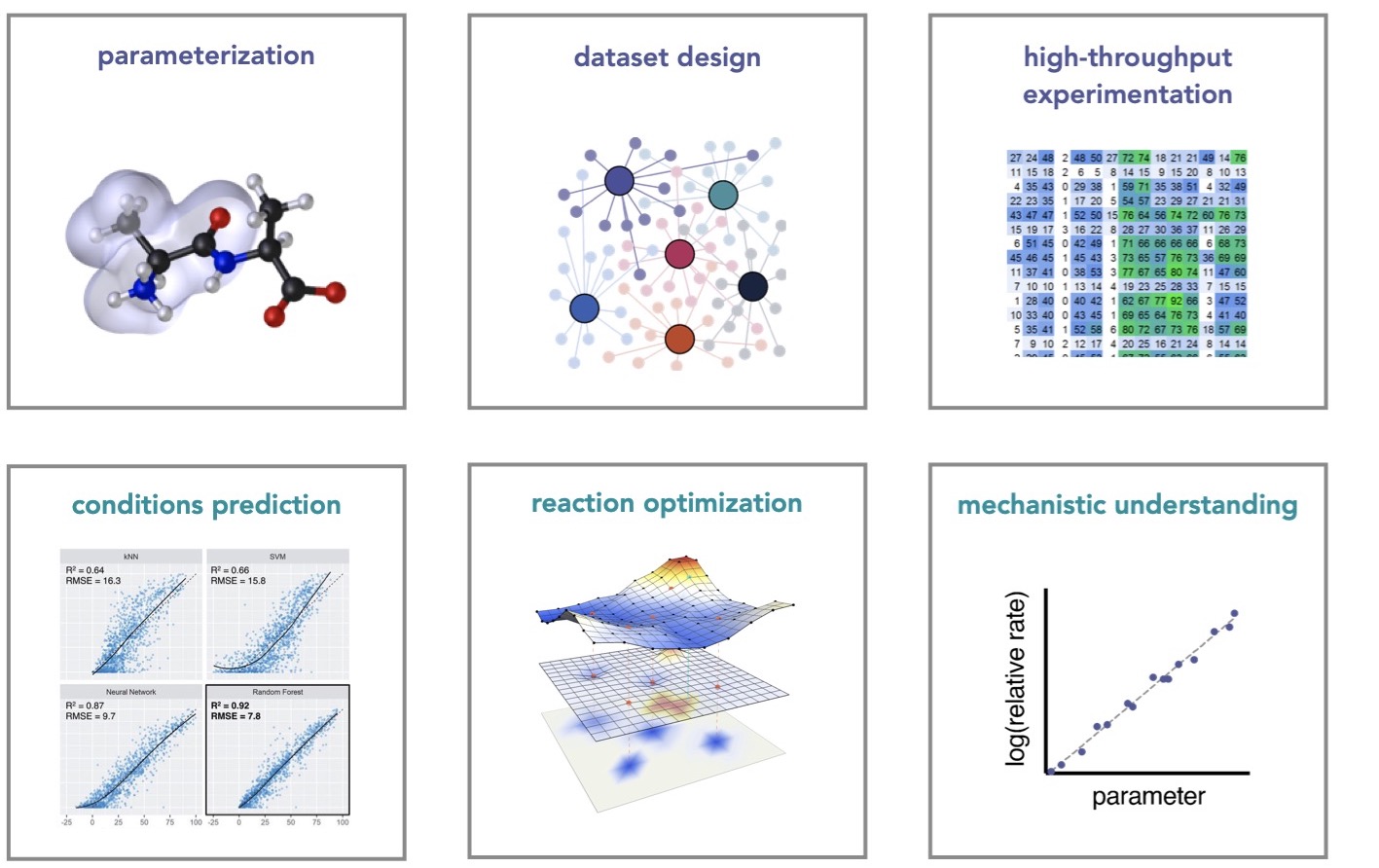
Reaction Condition Optimization
Optimization of a chemical reaction is a complex, multidimensional challenge that requires experts to evaluate various reaction parameters, including substrate, catalyst, reagent, additive, solvent, concentration, or temperature. However, due to the near infinite combinations of such conditions, it is only possible to evaluate a small subset of the condition space. Despite the recent advances in high throughput experimentation (HTE) and design of experiments (DOE) techniques, the optimized conditions are often limited by human bias and stagnation at a local maxima. Our lab has developed a framework for Experimental Design via Bayesian Optimization (EDBO) and an open-source software tool (www.edbowebapp.com) that allow chemists to easily integrate state-of-the-art optimization algorithms into their everyday laboratory practices. Overall, our studies suggest that adopting Bayesian optimization methods into everyday laboratory practices could facilitate more efficient synthesis of functional chemicals by enabling better-informed, data-driven decisions in future experimental work.
Data-Driven Mechanistic Interrogation
In our lab, interpretable models are deployed to gain mechanistic insight into a reaction. For example, decision trees were used to identify the %Vbur(min) threshold of the phosphine ligand that identifies reactivity in Suzuki-Miyaura coupling reactions. Furthermore, interpretable models including multivariate linear regression and dimensionality reduction were used to complement classical kinetic studies such as Eyring analysis and Hammett studies to interrogate how each descriptive feature of a molecule informs a reaction.
Reaction Performance Prediction
Predicting certain properties of a reaction can be valuable prior to experimental work by providing a platform to virtually screen substrates and suggest new reactions. In our lab, supervised learning methods including random forest, gaussian process regression, and neural networks were used to predict desired outcome of an organic reaction such as yield and selectivity of the product. Our ongoing efforts include low-data machine learning, optimization of general conditions, and multi-objective prediction methods.